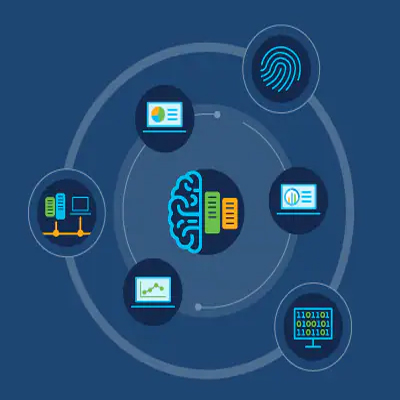
What is AIOps?
The phrase “AIOps,” or artificial intelligence for IT operations, was created by Gartner to refer to the use of artificial intelligence (AI) tools like machine learning models and natural language processing to automate and speed up operational processes.
Big data, analytics, and machine learning are specifically used by AIOps to carry out the following tasks:
- Gather and combine enormous volumes of data produced by various IT infrastructure elements, application requirements, performance-monitoring tools, and service ticketing systems.
- Intelligently distinguish “signals” from “noise” to identify significant occurrences and patterns related to application availability and performance issues.
- Identify the root causes and inform IT and DevOps about them for quick response and correction; in certain cases, these problems may be resolved automatically without human involvement.
By combining many disparate, manual IT operations technologies into a unified, intelligent, and automated IT operations platform, AIOps enables IT operations teams to react to slowdowns and disturbances more quickly—even preemptively.
It fills the gap between user expectations for little to no interruption in application performance and availability on the one hand, and a more varied, dynamic, and challenging-to-monitor IT environment and siloed teams on the other. The majority of experts concur that AIOps is the future of IT operations management, and as organizations place more emphasis on digital transformation initiatives, the need for AIOps will only increase.
Implementing AIOps
Each organization takes a different route to AIOps. Once you’ve established where you stand about achieving AIOps, you can start implementing solutions that let teams monitor, anticipate, and act quickly in response to operational IT issues. Look for the following qualities while evaluating technology to improve AIOps in your company:
- Observability: This term refers to software tools and practices for ingesting, aggregating, and analyzing a constant flow of statistical information from a distributed system and the hardware on which it runs to more effectively monitor, troubleshoot, and debug the application to satisfy customer experience standards, service level agreements (SLAs), and other business needs. Through data aggregation and consolidation, these solutions can provide a comprehensive picture of your applications, infrastructure, and network, but they do not take remedial action to address IT issues. They do not take remedial action to solve IT issues, but they do gather and aggregate IT data from several data sources across IT domains to inform end-users of possible difficulties, with the expectation that IT service teams will conduct the necessary remedy. While the data and visualizations generated by these tools are useful, they rely on IT companies to make judgments and respond effectively to technical concerns. In dynamic demand conditions, resource optimization that needs an operator to manually update operational systems may not yield the desired results.
- Predictive analytics: AIOps systems may analyze and correlate data to provide superior insights and automated actions, helping IT professionals to maintain control over increasingly complex IT environments while ensuring application performance. The ability to correlate and identify problems is a huge step forward for any IT Operations staff. It shortens the time it takes to find issues that would not have been discovered otherwise in the organization. Automatic anomaly detection, notifications, and solution recommendations will assist organizations, reducing total downtime as well as the number of issues and complaints. Predictive analytics may be used to automate dynamic resource optimization, which helps ensure application performance while securely reducing resource costs even when demand is very variable.
- Proactive response: Some AIOps systems will respond proactively to unforeseen occurrences like slowdowns and outages, bringing application performance and resource management together in real-time. They can uncover patterns and trends associated with various IT issues by putting application performance information into prediction algorithms. AIOps solutions, with the capacity to predict IT problems before they arise, may start relevant, automated processes in response, resolving difficulties rapidly. Intelligent automation will provide benefits to organizations such as improved mean time to discovery (MTTD).
This sort of technology is the future of IT operations management since it can assist businesses in improving both employee and consumer experiences. In addition to ensuring that IT service problems are resolved quickly, AIOps solutions also act as a safety net for IT operation teams by resolving problems that could be overlooked due to human oversight, such as organizational silos, under-resourced teams, and others.
Benefits
The key benefit of AIOps is that it speeds up the process of finding, managing, and fixing slowdowns and outages compared to manually sifting through alerts from various IT operations technologies. This has several significant repercussions:
- Faster mean time to resolution (MTTR): By sifting through IT operations noise and correlating operations data from various IT settings, AIOps can discover underlying causes and deliver cures faster and more precisely than humans. Organizations may now establish and exceed previously inconceivable MTTR targets. Vivy’s IT architecture, for example, lowered the mean time to repair (MTTR) for the company’s app by 66%, from three days to one day or less.
- Lower operating expenses: Automatic detection of operational difficulties and reprogrammed reaction scripts will lower operational costs, allowing for better resource allocation. This also frees up personnel resources to focus on more creative and difficult projects, resulting in a better employee experience. Providence saved over USD 2 million through optimization while ensuring app performance at peak periods.
- Improved observability and cooperation: AIOps monitoring tool integrations enable more efficient cross-team collaboration across DevOps, ITOps, governance, and security domains. Improved visibility, communication, and openness enable these teams to make better decisions and respond to challenges more rapidly. For example, Dealerware increased app performance during the pandemic and reduced delivery delays by 98 percent by adding additional observability to their container-based design.
- Make the transition from reactive to proactive to predictive management: With built-in predictive analytics capabilities, AIOps constantly learn to detect and prioritize the most critical alerts, allowing IT teams to resolve potential issues before they cause slowdowns or disruptions. Electrolux reduced the mean time to detect (MTTD) for IT issues from three weeks to one hour and saved over 1,000 hours per year by automating repair operations.
Use cases
AIOps combines big data, sophisticated analytics, and machine learning to address the following use cases:
- Root cause analysis: As the term implies, root cause analyses look for the underlying causes of problems to pinpoint the best remedies. Focusing on the root causes of the issue rather than just the symptoms may help teams save money on labor. An AIOps platform, for example, may identify the main reason for a network failure and put security measures in place to make sure it doesn’t happen again.
- Finding anomalies in a dataset: AIOps tools can comb through vast amounts of historical data. These irregularities act as “signals,” spotting and foretelling risky circumstances like data breaches. With this ability, businesses may prevent costly outcomes including negative public perception, regulatory fines, and dwindling consumer trust.
- Performance Monitoring: Because modern applications are sometimes separated by numerous levels of abstraction, it can be difficult to determine which underlying physical server, storage, and networking resources are powering particular applications. AIOps aid in bridging this gap. It monitors cloud infrastructure, virtualization, and storage systems, reporting on parameters including consumption, availability, and response times. Furthermore, it takes advantage of event correlation capabilities to consolidate and aggregate information, allowing for improved information consumption by end-users.
- Cloud adoption/migration: Most firms embrace cloud gradually, rather than all at once, resulting in a hybrid multi-cloud system (private cloud, public cloud, multiple suppliers) with many interdependencies that might change too fast and often to document. AIOps may drastically minimize the operational risks of cloud migration and hybrid cloud approaches by offering clear visibility into these interdependencies.
- Adoption of DevOps: DevOps accelerates development by empowering development teams to deploy and modify infrastructure, but IT must still maintain that infrastructure. AIOps delivers the visibility and automation required by IT to assist DevOps without requiring significant additional management work.
How do AIOps operate?
The simplest approach to understanding how AIOps works is to look at how each AIOps component technology—big data, machine learning, and automation—plays a part in the process.
AIOps employs a big data platform to centralize siloed IT operations data, people, and technologies. This information may contain the following:
- Historical performance and event information
- Streaming real-time operations events
- System logs and metrics
- Network data, including packet data
- Incident data and ticketing
- Application demand data
- Infrastructure data
Then, AIOps employs targeted analytics and machine learning capabilities:
- Distinguish major event alerts from ‘noise’: AIOps crawls through your IT operations data to extract signals—important abnormal event warnings—from noise (everything else).
- Determine the underlying reasons and provide solutions: AIOps can correlate anomalous events with other event data across environments to pinpoint the source of an outage or performance issue and provide solutions.
- Automate responses, including real-time proactive resolution: At the very least, AIOps may route alarms and proposed solutions to the relevant IT teams, or even form response teams based on the nature of the problem and the solution. It can analyze the results of machine learning in a variety of situations to set off autonomous system responses that resolve issues in real-time even before people are aware they exist.
- Constantly learn to better management of future problems: AI models may also assist the system in learning about and adapting to environmental changes, such as new infrastructure provided or reconfigured by DevOps teams.
IBM and AIOps
Investigate IBM’s AIOps and IT Automation portfolio. IBM AIOps assists enterprises in ensuring app performance while securely reducing IT expenditures. Organizations have been able to cut IT costs by half, save up to $2 million in incident management, and cut MTTR by half. Furthermore, teams were able to debug apps 75% quicker.
- This Omdia Universe guide (PDF, 3.7 MB) can help you better understand how to choose an AIOps solution.
- Learn more about the state of AIOps by reading this EMA market research study provided by IBM.
- IBM® Turbonomic® Application Resource Management integrates with your existing IT operations solutions, connects siloed teams and data, and transforms manual, reactive procedures into continuous application resource optimization while securely decreasing cloud use by 33%. Check out the Total Economic ImpactTM research.
- IBM Cloud Pak® for Watson AIOps integrates with your existing toolchain to provide proactive incident management and automated remediation, reducing customer-facing outages by up to 50% and mean time to recovery (MTTR) by up to 50%. To understand more, read the Total Economic ImpactTM research.
- With IBM® Observability by Instana APM, your applications may be more responsive to user demands. Accelerate CI/CD pipelines to deliver applications quicker and at a lower cost by providing completely automated application observability and context required for intelligent actions and application performance.